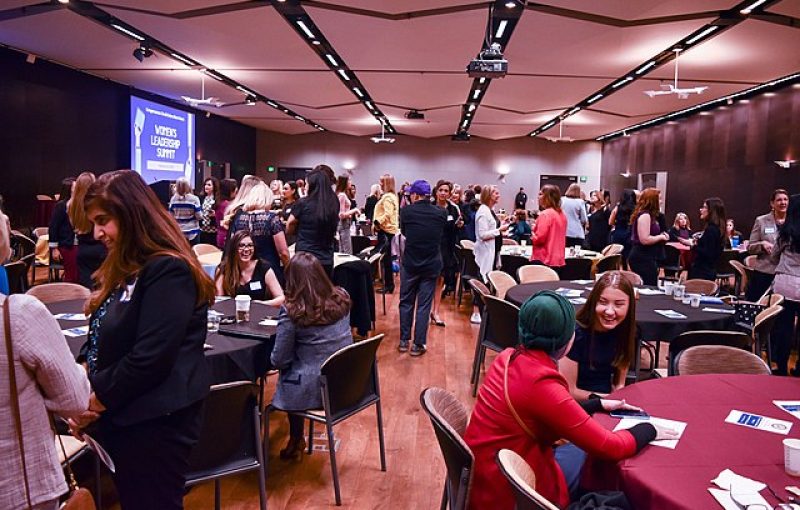
Using AI to sort through evaluation research
by Jon Scaccia December 16, 2021Let’s talk evaluation. I haven’t done a summary of evaluation in a long time, and since we’re dialing up a really big project with our friends at RISE, it’s as good a time as any to see what’s been going on in the evaluation field.
I would hypothesize that the ratio of evaluation practitioners to evaluation researchers is something like 10:90. It’s also likely that these practitioners don’t often dive into the literature.
For the past few years, I’ve been utilizing AI to cluster and summarize a big number of scientific studies in order to make a better way to extract the essentials. Without further ado, here are the themes and trends of the last 31 days of evaluation research.
I started by pulling all articles from the following journals.
- Evaluation
- Evaluation and the Health Professions
- Evaluation and Program Planning
- Evaluation Review
- Research Evaluation
- The Canadian Journal of Program Evaluation
- The American Journal of Evaluation
- The Journal of Multidisciplinary Evaluation
- New Directions for Evaluation
However, I encountered two issues. Only three of these journals have been updated in the previous thirty days. So I then attempted to gather everything that mentioned “evaluation.” This was a big mistake. That method gathered material from diverse disciplines unrelated to “program evaluation.”
However, we still had a nice number–140 total articles from three journals (though 131 of those were from Evaluation and Program Planning.)
Bag-of-Words
Bag-of-words was my first approach. This is a technique that most individuals are acquainted with. It tallies the frequency of words in a collection of documents. I decided to depict it as a word cloud, as shown in the figure above. It’s also fascinating to notice how many times health and implementation appear in this graph!
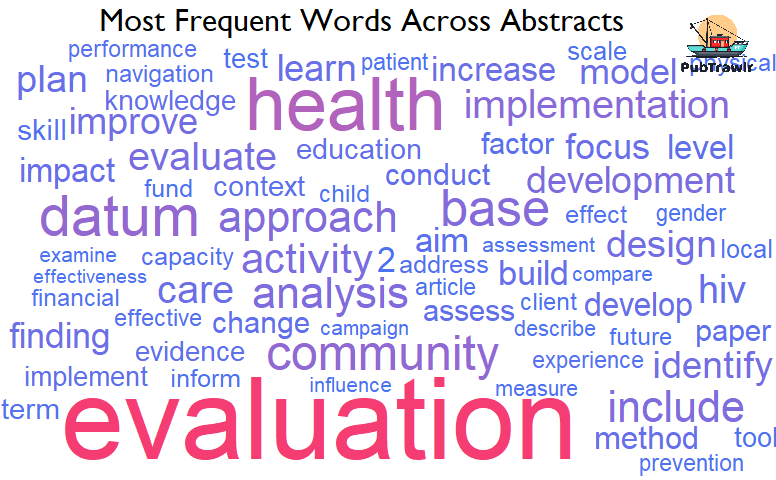
To go even further, I constructed a network plot to reveal word strings. This means that we may trace the pathways to discover sequences of words. In this plot, the larger the circle represents a more frequent appearance. A thicker line indicates a greater frequency of occurrence for each pair.
In this figure, we see that health, program, and evaluation are our most central nodes. But, we can also see interesting topics, like human trafficking, citizen empowerment, storytelling, and campaign messaging.
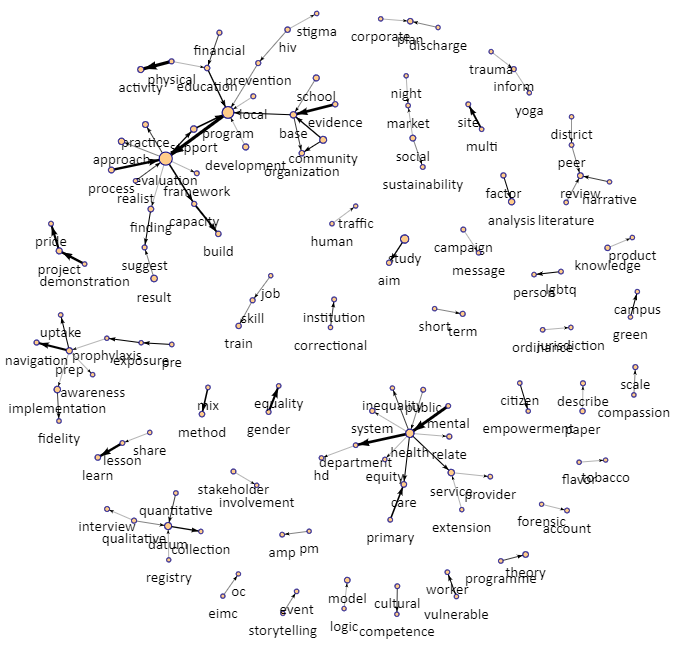
Topic Modeling Evaluation Articles
Let’s look at topic modeling in further detail. This is a method of determining the clusters of terms (topics) that appear across our abstracts. The most frequent topics are shown in the figure above, with the most frequent words to their left. Finally, we utilize the MeSH terms for these clusters to define them. This is a controlled vocabulary employed by NLM to assist maintain a hierarchical knowledge structure.
Over that past month, we can see that 18 articles talked about social adjustment, communication, and learning. We also see a number of articles about gender identity and roles.
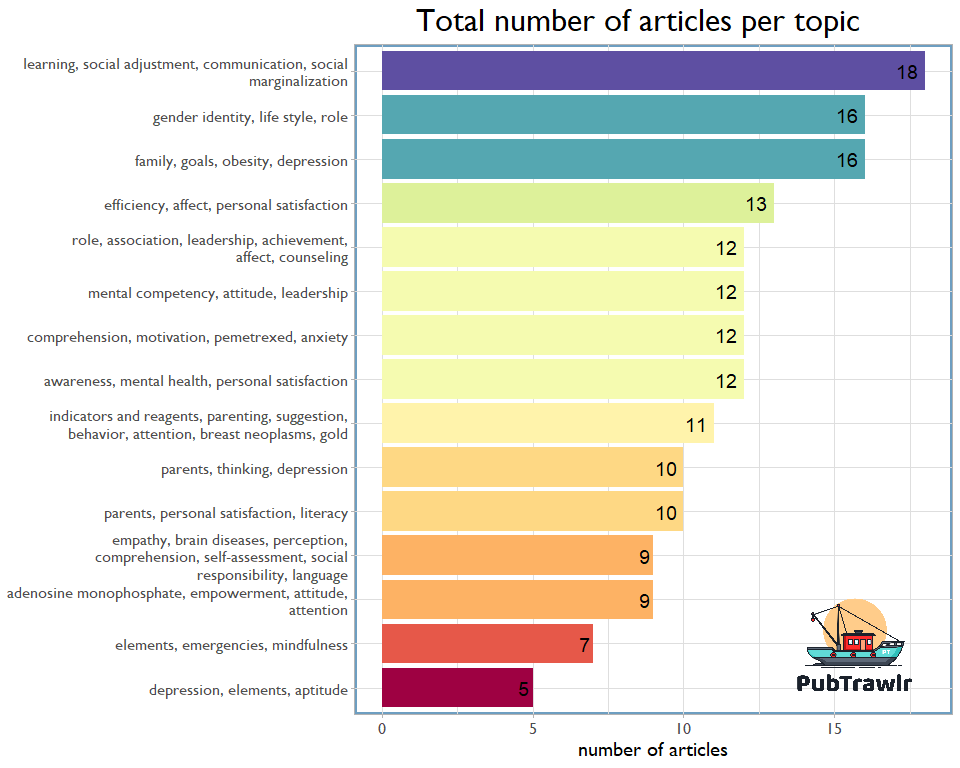
I next created a correlation matrix to show how the material may be linked. In this graph, the topics are the nodes, with the lines between them representing the articles’ relationships. It should come as no surprise that the parents, thinking, depression topic was strongly related to depression, elements, and aptitude.
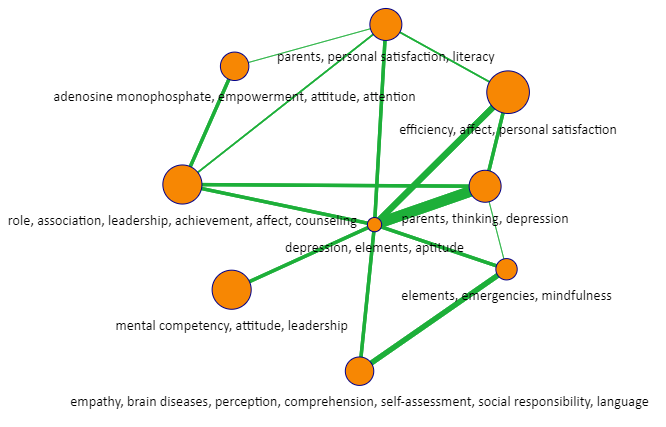
Let’s add one more thing that might be helpful. In the attached file, I found the article that was most representative of these topics and generated a summary of the topic.
Finally, and somewhat experimentally, I trained a list of word vectors, then plotted the topic 40 words. This shows how the terms are related to one another within this set of 140 articles. Plan and theory are closely related to one another, as are focus and activity.
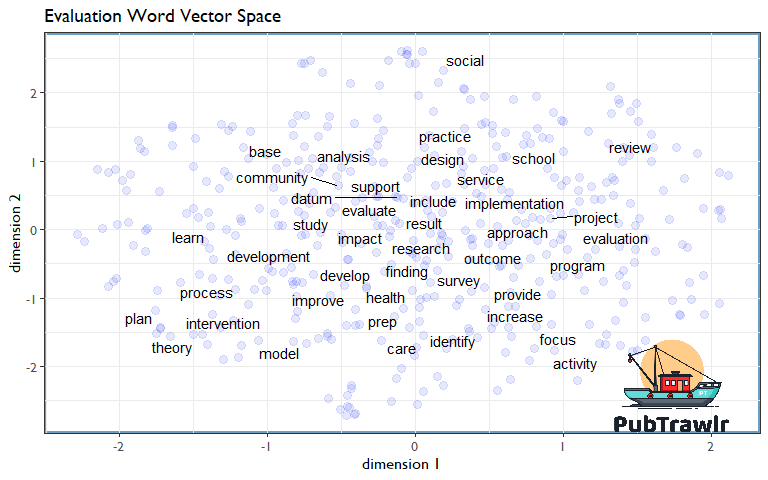
Summing it all up
I hope that this blog post has helped you understand how AI can be used to sort through evaluation research and find patterns in the data. If you want more information on what we do, check out our website or contact us directly for a consultation! We’re happy to help others use these technologies to increase their productivity and efficiency when it comes to research.
Leave a Reply